We propose a new and completely data-driven approach for generating a photo-consistent image transform. We show that simple classical algorithms which operate in the transform domain become extremely resilient to illumination changes. This considerably improves matching accuracy, outperforming the use of state-of-the-art invariant representations as well as new matching methods based on deep features. The transform is obtained by training a neural network with a specialized triplet loss, designed to emphasize actual scene changes while attenuating illumination changes. The transform yields an illumination invariant representation, structured as an image map, which is highly flexible and can be easily used for various tasks. We point out that the utility of our method is not restricted to handling illumination invariance, and that it may be applied for generating representations which are invariant to additional types of nuisance, undesired, image variants.
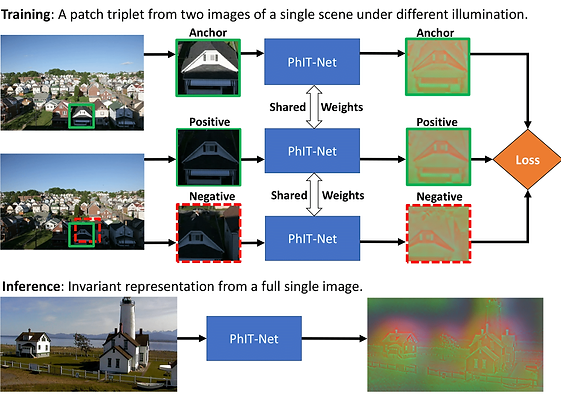
Paper (D. Kaliroff, G. Gilboa, arXiv 1911.12641 )
Graduate seminar presentation (PDF)
Graduate seminar recording (Youtube)